GPU如何訓(xùn)練大批量模型?方法在這里
擴展到極致
本文引用地址:http://www.biyoush.com/article/201810/393173.htm你可以在 GPU 上訓(xùn)練連一個樣本都無法加載的模型嗎?
如果你的架構(gòu)沒有太多跳過連接,這就是可能的!解決方案是使用梯度檢查點(gradient-checkpointing)來節(jié)省計算資源。
基本思路是沿著模型將梯度在小組件中進行反向傳播,以額外的前饋傳遞為代價,節(jié)約存儲完整的反向傳播圖的內(nèi)存。這個方法比較慢,因為我們需要添加額外的計算來減少內(nèi)存要求,但在某些設(shè)置中挺有意思,比如在非常長的序列上訓(xùn)練 RNN 模型(示例參見 https://medium.com/huggingface/from-zero-to-research-an-introduction-to-meta-learning-8e16e677f78a)。
這里不再贅述,讀者可以查看以下鏈接:
TensorFlow:https://github.com/openai/gradient-checkpointing
PyTorch 文檔:https://pytorch.org/docs/stable/checkpoint.html
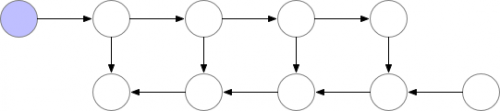
「節(jié)約內(nèi)存」(Memory-poor)策略需要 O(1) 的內(nèi)存(但是要求 O(n2) 的計算步)。
充分利用多 GPU 機器
現(xiàn)在我們具體來看如何在多 GPU 上訓(xùn)練模型。
在多 GPU 服務(wù)器上訓(xùn)練 PyTorch 模型的首選策略是使用 torch.nn.DataParallel。該容器可以在多個指定設(shè)備上分割輸入,按照批維度(batch dimension)分割,從而實現(xiàn)模塊應(yīng)用的并行化。
DataParallel 非常容易使用,我們只需添加一行來封裝模型:
parallel_model = torch.nn.DataParallel(model) # Encapsulate the model
predictions = parallel_model(inputs) # Forward pass on multi-GPUs
loss = loss_function(predictions, labels) # Compute loss function
loss.backward() # Backward pass
optimizer.step() # Optimizer step
predictions = parallel_model(inputs) # Forward pass with new parameters
但是,DataParallel 有一個問題:GPU 使用不均衡。
在一些設(shè)置下,GPU-1 會比其他 GPU 使用率高得多。
這個問題從何而來呢?下圖很好地解釋了 DataParallel 的行為:
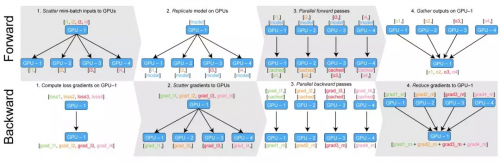
使用 torch.nn.DataParallel 的前向和后向傳播。
在前向傳播的第四步(右上),所有并行計算的結(jié)果都聚集在 GPU-1 上。這對很多分類問題來說是件好事,但如果你在大批量上訓(xùn)練語言模型時,這就會成為問題。
我們可以快速計算語言模型輸出的大?。?/p>

語言模型輸出中的元素數(shù)量。
假設(shè)我們的數(shù)據(jù)集有 4 萬詞匯,每一條序列有 250 個 token、每個 batch 中有 32 條序列,那么序列中的每一個元素需要 4 個字節(jié)的內(nèi)存空間,模型的輸出大概為 1.2GB。要儲存相關(guān)的梯度張量,我們就需要把這個內(nèi)存翻倍,因此我們的模型輸出需要 2.4GB 的內(nèi)存。
這是典型 10GB GPU 內(nèi)存的主要部分,意味著相對于其它 GPU,GPU - 1 會被過度使用,從而限制了并行化的效果。
如果不調(diào)整模型和/或優(yōu)化方案,我們就無法輕易減少輸出中的元素數(shù)量。但我們可以確保內(nèi)存負載在 GPU 中更均勻地分布。
多 GPU 機器上的均衡負載
解決辦法是把每部分輸出保留在其 GPU 上,而不是將它們聚集到 GPU-1 上。我們也需要分配損失標(biāo)準(zhǔn)計算,計算損失并進行反向傳播。
幸而,張航開源了一個名為 PyTorch-Encoding 的 PyTorch 包,它包含了這些定制的并行化功能。
我提取并稍稍改動了這個模塊,你可以從以下地址下載 gist(parallel.py)來納入并調(diào)用你的代碼。它主要包括兩個模塊:DataParallelModel 和 DataParallelCriterion,它們的用途如下:
下載地址:https://gist.github.com/thomwolf/7e2407fbd5945f07821adae3d9fd1312
from parallel import DataParallelModel, DataParallelCriterion
parallel_model = DataParallelModel(model) # Encapsulate the model
parallel_loss = DataParallelCriterion(loss_function) # Encapsulate the loss function
predictions = parallel_model(inputs) # Parallel forward pass
# "predictions" is a tuple of n_gpu tensors
loss = parallel_loss(predictions, labels) # Compute loss function in parallel
loss.backward() # Backward pass
optimizer.step() # Optimizer step
predictions = parallel_model(inputs) # Parallel forward pass with new parameters
DataParallelModel 和 torch.nn.DataParallel 的區(qū)別在于,前向傳播的輸出(predictions)沒有聚集在 GPU-1 上,而是作為 n_gpu 張量的元組,每個張量分布在相應(yīng)的 GPU 上。
DataParallelCriterion 容器封裝了損失函數(shù),并把 n_gpu 張量元組和目標(biāo)標(biāo)簽張量作為輸入。它在每個 GPU 上并行計算損失函數(shù),像 DataParallel 分割模型輸入一樣分割目標(biāo)標(biāo)簽張量。
下圖說明了 DataParallelModel/DataParallelCriterion 的內(nèi)部情況:
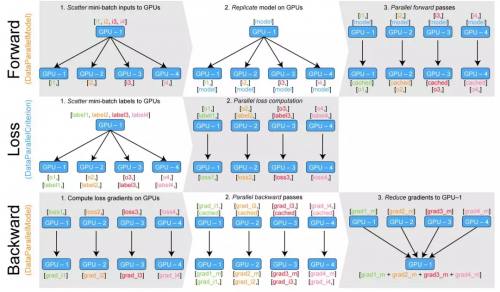
使用 DataParallelModel 和 DataParallelCriterion。
以下是你可能會遇到的兩個特定案例的解決辦法:
你的模型輸出幾個張量:你可能想分解它們:output_1, output_2 = zip(*predictions)
有時候你并不想使用并行損失函數(shù):收集 CPU 上的所有張量:gathered_predictions = parallel.gather(predictions)
評論